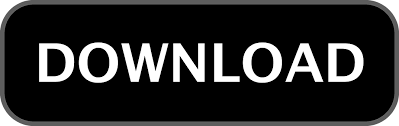
An important policy question is whether Medicaid expansions will decrease ER utilization and increase primary care visits by the previously uninsured. We are particularly interested in measuring the effect of Medicaid on emergency room (ER) use because it is the main delivery system through which the uninsured receive health care, and the uninsured could potentially receive higher quality health care through primary care visits. We apply the PATT-C estimator to measure the effect of Medicaid coverage on health care use for a target population of adults who may benefit from government-backed expansions to the Medicaid program. By explicitly modeling compliance, our approach allows researchers to decompose population estimates by covariate group, which is useful for policymakers in evaluating the efficacy of policy interventions for subgroups of interest in a population. However, we do not observe the population compliance rate, and it is likely to differ across subgroups based on pretreatment covariates. When extrapolating RCT results to a population, one might simply reweight the ITT effect according to the covariate distribution of the population and then divide by the proportion of treated compliers in the population in order to yield a population average effect of treatment on treated compliers. When estimating the average causal effect for compliers from an RCT, researchers typically scale the estimated ITT effect by the compliance rate, assuming that there is only single crossover from treatment to control. In our approach, the potential outcomes between the complier treated and complier controls are not exchangeable by design, since we need to assume we know the compliance model. Reweighting methods typically leverage exchangeability of potential outcomes between the covariate-adjusted treated and controls in the RCT. propose a method of reweighting the responses of individuals in an RCT according to the covariate distribution of the population. Individuals in the RCT and population are then weighted according to the inverse of the estimated propensity score. , for example, a propensity score model is used to predict participation in the RCT, given pretreatment covariates common to both the RCT and population data. Our approach for estimating PATT-C differs from previous reweighting methods because we only need to estimate the potential outcomes for RCT compliers and we cannot observe who in the control group would have complied had they been assigned treatment. Assuming that the response surface is the same for compliers in the RCT and population members who received treatment, we then predict the response surface for all RCT compliers and use the predicted values from the response surface model to estimate the potential outcomes of population members who received treatment, given their covariates. We model compliance in the RCT in order to predict the likely compliers in the RCT control group. We propose a reweighting method for estimating complier–average causal effects for the target population from RCT data with noncompliance, and refer to this estimator as the Population Average Treatment Effect on Treated Compliers (PATT-C). Noncompliance biases the intention–to–treat (ITT) estimate of the effect of treatment assignment toward zero. For individuals assigned to control, we are unable to observe who would have complied had they been assigned treatment. Noncompliance occurs when individuals who are assigned to the treatment group do not accept the treatment.
#AVERAGE TREATMENT EFFECT ON THE TREATED FULL#
Existing approaches to this problem are based in settings where there is full compliance with treatment however, noncompliance is a prevalent issue in RCTs. External validity is particularly relevant to policymakers who require information on how the treatment effect would generalize to the broader population.Ī new research frontier in causal inference focuses on developing methods for extrapolating RCT results to a population. For example, participants in an RCT in which individuals volunteer to sign up for health insurance may be in poorer health at baseline than the overall population. An RCT may give unbiased estimates of sample average treatment effects, but external validity is an issue when RCT participants are unrepresentative of the actual population of interest. Randomized control trials (RCTs) are the gold standard for estimating the causal effect of a treatment.
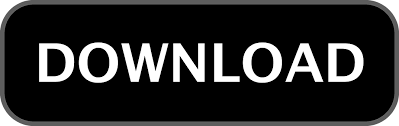